=Overview
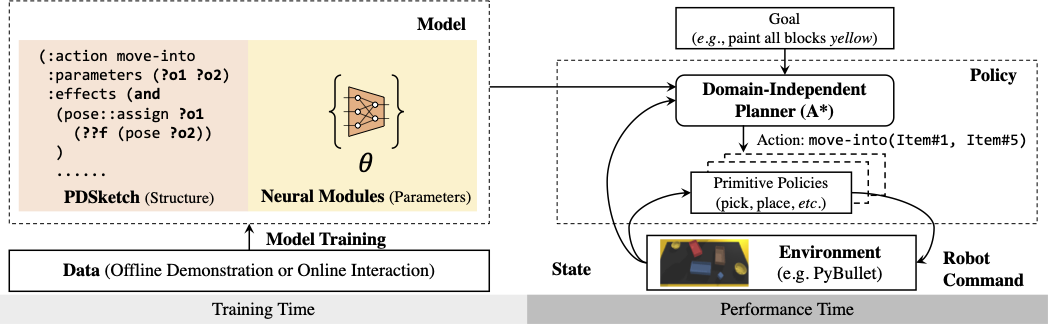
Figure 1: The life cycle of a PDSketch model. A PDSketch model is composed of a model structure definition and a collection of trainable neural modules. The model parameters can be learned from data. During performance time, the model is used by a domain-independent planner to form a policy that directly interacts with the environment.
Abstract: This paper studies a model learning and online planning approach towards building flexible and general robots. Specifically, we investigate how to exploit the locality and sparsity structures in the underlying environmental transition model to improve model generalization, data-efficiency, and runtime-efficiency. We present a new domain definition language, named PDSketch. It allows users to flexibly define high-level structures in the transition models, such as object and feature dependencies, in a way similar to how programmers use TensorFlow or PyTorch to specify kernel sizes and hidden dimensions of a convolutional neural network. The details of the transition model will be filled in by trainable neural networks. Based on the defined structures and learned parameters, PDSketch automatically generates domain-independent planning heuristics without additional training. The derived heuristics accelerate the performance-time planning for novel goals.
=Resources
- The original version in [PyTorch (Alpha)].
- [Talk slides] presented at NeurIPS 2023.